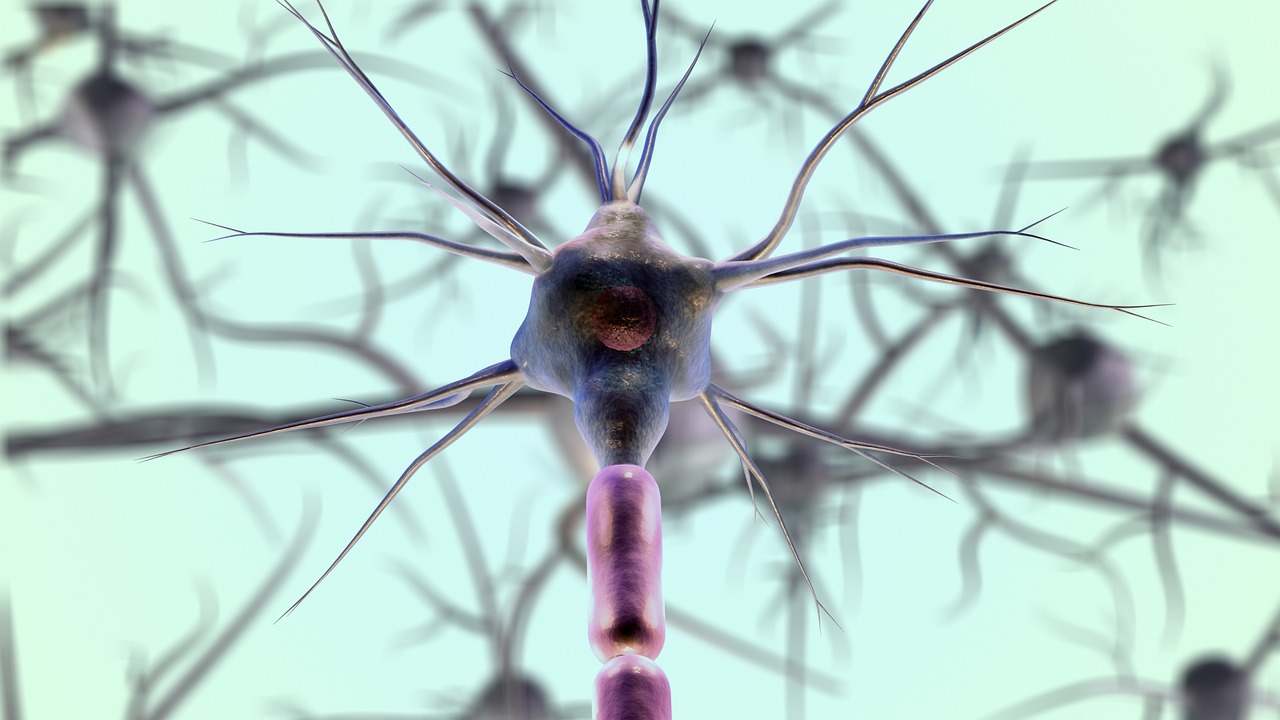
Background
Neurodegenerative disorders, such as Alzheimer's disease (AD), Parkinson's disease (PD), and dementia with Lewy bodies, are a worldwide health issue due to their wide range of clinical symptoms and complicated comorbidities.
Current research struggles to acquire complete clinical data, which limits statistical designs. Innovative data-driven strategies that use large autopsy cohorts are required to improve diagnosis.
Brain banks give vital information on neurodegenerative illnesses, but drawbacks such as limited clinical data and binary case-control designs impede development.
About the study
In the present study, researchers created a computer pipeline to translate medical record summaries from Netherlands Brain Bank (NBB) donors into clinical illness trajectories, which included 84 neuropsychiatric symptoms and signs recognized using natural language processing.
They scanned NBB donor files, defined and predicted clinical features in the recorded history, translated predicted symptoms and signs into clinical illness trajectories, and applied them for downstream analysis.
The researchers developed a novel cross-disorder clinical classification system including 90 neuropsychiatric symptoms and signs related to brain illnesses and general well-being. One scorer evaluated 18,917 phrases from a randomly selected group of 293 contributors to build a dataset for refining, validating, and testing various Natural Language Processing (NLP) models.
The researchers optimized five model designs [support vector machine (SVM), bag of words (BOW), T5, PubMedBERT, and Bio_ClinicalBERT] and chose the best one based on microprecision.
The team developed the clinical illness trajectories, including several neuropsychiatric symptoms and signs, duration, and more donors than previously published. They then ran an enrichment assessment to investigate whether the estimated clinical features were more prevalent in each disease than expected.
To assess the diagnostic accuracy of this brain autopsy cohort, the researchers cleaned and matched CD descriptions to the human disease ontology and compared the generated clinical diagnosis labels to the neurodegenerative diagnoses.